Executive Summary
Laboratory information systems (LIS) are a critical component of national health information systems architectures. Clinicians, lab professionals, national health sector leaders, and the donor community look to lab data to improve patient care and treatment, assist laboratories in achieving accreditation, support disease surveillance efforts, and inform outbreak responses. As low and middle income countries progress in development of coordinated eHealth investments, LIS can be leveraged to integrate with other clinical information systems and with disease surveillance and reporting systems to meet public health goals.
The primary open-source product which fills the distinct use cases and workflows of reference labs is OpenELIS (https://sites.google.com/site/openelisglobal/). OpenELIS was originally developed in several state public health laboratories within the US, and has since been adapted and deployed in Vietnam, Haiti, and Cote d’Ivoire at wide scale, and has also been integrated into and deployed with the leading OpenMRS distribution, Bahmni. Key features available within OpenELIS include: flexible test catalogue management; batch processing of bio-samples; barcode generation and printing capability; “plug and play” integration with automated analyzers covering a wide range of test types; integration with a data visualization dashboard to display indicators for national surveillance; generation of CSV file exports to facilitate flexible indicator reporting to a variety of stakeholders; and language localization in English and French.
This proposal will integrate the OpenELIS open-source LIS (https://sites.google.com/site/openelisglobal/) with the OpenMRS electronic medical record (EMR) (https://openmrs.org/), with the integrated Bahmni clinical information system package (https://www.bahmni.org/), as well as with the OpenLMIS logistics management information system (LMIS) (http://openlmis.org/). The project will advance interoperability of OpenELIS with other systems in the context of both direct bridges as well as linkage through heatlh information exchange (HIE), modeled on the OpenHIE framework (https://ohie.org/). The proposed project will significantly advance flexible, standardized, deployable solutions for interoperability of OpenELIS with leading EMR and LMIS products, thereby improving the ability of the laboratory sector in LMICs to support laboratory monitoring for patient care and outbreak detection.
Consortium Team
The consortium team is led by the University of Washington (UW) and includes Village Reach, and Bahmni Coalition. There are two groups from UW which will come together to carry out this proposal: I-TECH (https://www.go2itech.org/) and the UW Clinical Informatics Research Group (CIRG) (http://cirg.washington.edu/). I-TECH is a Center within the UW Department of Global Health (DGH) that leads health systems strengthening initiatives in more than 20 countries. I-TECH has led OpenELIS development and implementation in Haiti and Cote d’Ivoire since 2009 and 2010 respectively. In Haiti and Cote d’Ivoire, I-TECH has supported implementation of OpenELIS in more than 75 national public health reference labs as well as in large-volume clinical laboratories. As a part of the Haiti project, I-TECH’s Haiti-based software development and implementation team has collaborated with the international IT firm Soldevelo (https://www.soldevelo.com/) to establish integration between OpenELIS and OpenMRS, as well as an OpenHIE-based interoperability layer which is suitable for supporting data exchange between LIS and EMRs as well as between LIS and the DHIS2-based national aggregate data reporting system.
As of October 2018, I-TECH is supporting the launch of a unified Global Health Information Systems (GHIS) Unit, which will serve as a central hub within I-TECH and the UW DGH for health informatics expertise, under the leadership of faculty member Dr. Nancy Puttkammer. The GHIS Unit brings together experienced I-TECH staff from separate country teams with a range of expertise relevant for health informatics in global settings, including in requirements gathering and technical design, software development, implementation planning, technology project management, human capacity building and training, data analytics, and assessment and evaluation of digital health solutions. The GHIS Unit is set up as a distinct business unit with a flexible mechanism for contracting technical assistance services to serve digital health project needs for Principal Investigators (PIs) from across UW, or to serve clients outside of UW. This mechanism offers the potential to harness expertise from faculty, staff, and students from the UW’s Schools and Departments including Health Sciences, Computer Science and Engineering, Bioengineering, Information Sciences, Business and others.
I-TECH also brings to the project the expertise in laboratory systems in LMIC, through our Laboratory Systems Strengthening (LSS) Team. Led by Dr. Lucy Perrone, a public health laboratory advisor specializing in infectious disease diagnosis, surveillance and response, and laboratory capacity building in LMICs, the team leverages partnerships within UW and with external collaborators globally on supporting laboratory capacity building. The team’s mission is to improve laboratory operations for optimal patient care and treatment, disease surveillance and response, and biosecurity. The team has conducted training and mentoring in laboratory leadership and management, supported policy development for laboratories, and worked with reference and clinical laboratories on advancement toward accreditation. As part of reinforcing good laboratory practice, the team has also supported customization and implementation of LIS for improved information management within the laboratory. The LSS team is available to contribute expertise in the fit between LIS and laboratory workflows and systems to the proposed project.
As noted, the UW team also includes CIRG, under leadership from Jan Flowers. CIRG designs, develops, builds, and operates information systems that securely manage health information for projects in clinical, public, and global health settings. CIRG has led numerous lab informatics projects involving OpenELIS and BLIS, founded OpenLabConnect, and developed LIS interoperability projects, and has worked in Haiti, Cote d’Ivoire, Kenya, Mozambique, Cameroon, Namibia, and Vietnam. Ms. Flowers serves on the board of directors for both OpenELIS Foundation and OpenMRS, and are the founders and leads the OpenHIE LIS Community of Practice (hereafter referred to as the “LIS COP”), which was funded under Digital Square Notice B to develop and share common standards and best practices amongst the open-source LIS community.
Village Reach (http://www.villagereach.org) works with Ministries of Health to solve healthcare delivery challenges in low-resource environments. To do this, it focuses on being is a global health informatics innovator in logistics management that develops, tests, implements and scales new solutions to critical health system challenges in low-resource environments. Village Reach leads the development of the founders and leaders of OpenLMIS, the leading logistics management information system in LMIC. Mary Jo Kochendorfer will be the point person representing OpenLMIS in this project.
The Bahmni Coalition (https://www.bahmni.org/bahmni-coalition/) is a group of organizations leading and governing the Bahmni software, an OpenMRS distribution that specifically addresses the use case of hospital management. The Coalition includes organizations which use Bahmni, contribute to Bahmni development or implementation, or offer services around Bahmni.
Resumes for key staff from I-TECH’s GHIS Unit, CIRG, VillageReach, and Bahmni are included as attachments to the final proposal.
Project Description
Problem Statement
Strong laboratory systems are integral for diverse public health purposes such as monitoring suppression of HIV viral load among patients receiving antiretroviral treatment, measuring antimicrobial resistance of pathogens, and detecting outbreaks of cholera and other infectious diseases. LIS systems can support good laboratory practice. Interoperability between LIS, EMR, and LMIS can advance global public health goals; however, there are not currently standards-based integrations between leading open-source products for each of these system types. LIS and EMR interoperability can substantially impact patient care by improving the accuracy of orders and results, reduce effort of clinical and lab staff in data entry, and reduce turnaround times for providing test results to clinicians, patients, and public health decision makers. LIS and LMIS interoperability can improve quantification estimates for lab reagents, test kits, and other consumables within national supply chains, as well as improve decision making by laboratory personnel about which tests should be run at which laboratories, based on stock levels for key lab commodities. HIS ecosystems in low- and middle-income countries can be substantially strengthened by improved integration of leading LIS, EMR, and LMIS products.
Demonstrated Need and Potential for Health Impact
LIS are a critical component of national health information systems architectures. Clinicians, laboratory professionals, national health sector leaders, and the donor community look to laboratory data to improve patient care and treatment, assist laboratories in achieving accreditation, and support disease surveillance efforts and inform outbreak responses. First, LIS play an integral role in laboratory quality management (ISO 15189) by improving organization and management of bio-samples and testing queues, by reducing transcription errors in laboratory results, and by reducing turnaround-time for diagnostic test results. Second, LIS can facilitate a critical link between the laboratory and clinical services, so that patients can be appropriately diagnosed and managed in light of accurate and timely laboratory test results. Finally, LIS can contribute to disease surveillance by improving the availability of laboratory data at regional and national levels. As low and middle income countries progress in development of coordinated eHealth investments, LIS can be leveraged to integrate with other clinical information systems and with disease surveillance and reporting systems.
Presently, OpenELIS is a leading open-source product with particular value for use in high-volume reference laboratories. Effective national laboratory systems typically include clinical laboratories as well as reference laboratories at regional or national levels. Reference laboratories have distinct information management needs, such as the need to manage a large catalogue of test options, to handle batch processing of samples, to run test samples for quality control, and to receive orders and dispatch results to lower-level laboratories at high volume.
There are several existing open-source LIS products focused on smaller clinical laboratories, such as Basic Laboratory Information System (BLIS) and SENAITE Labs (formerly Bika Labs). These systems are optimized for the workflows of basic clinical labs operating within clinics and smaller hospitals, where a limited array of laboratory test types and a limited volume of tests are run. These systems can play complementary roles to OpenELIS within national laboratory systems.
In contrast, OpenELIS fills the distinct use cases and workflows of reference labs. OpenELIS features such as batch processing, barcode generation, and analyzer integration provide laboratories processing high sample volumes with a means of reducing turnaround time. The value of OpenELIS in LMICs is demonstrated by the persistent use of the product in more than 20 high-volume regional laboratories in Haiti more than 18 months after withdrawal of external donor funding. It is also illustrated in Cote d’Ivoire, where the Ministry of Health has shown its commitment to the OpenELIS product by expanding its use in regional labs over an alternative open-source LIS.
The proposed project will significantly advance flexible, standardized, deployable solutions for interoperability of OpenELIS with leading EMR and LMIS products, thereby improving the ability of the laboratory sector in LMICs to support laboratory monitoring for patient care and outbreak detection.
Technical Approach
Work Stream 1: Integrating OpenELIS and OpenMRS
OpenELIS and OpenMRS are the two leading open-source systems in the LIS/EMR technology space for LMIC, and as such, cover a significant portion of implementations. The consortium team will seek to design and develop minimum viable products (MVPs) for two strategies for linking OpenELIS and OpenMRS using the following two approaches:
- Direct Bridge: This assumes that the systems are hosted on a co-located server and involves a direct exchange of laboratory orders and laboratory results data between the systems for a single health facility. This solution would be specifically for implementers who had no ability to support or need for a larger architecture to address additional or more advanced interoperability use cases. A technical description of this strategy is shown in Figure 1 and a schematic is shown in Figure 2.
Figure 1: Technical Description of OpenELIS – OpenMRS Direct Bridge
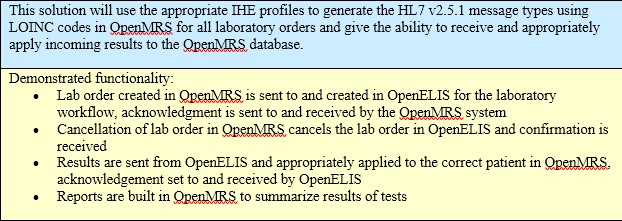
Figure 2: System Architecture for OpenELIS - OpenMRS Interoperability*
*Note: “iSantéPlus” is Haiti’s national electronic medical record system, built on the OpenMRS platform
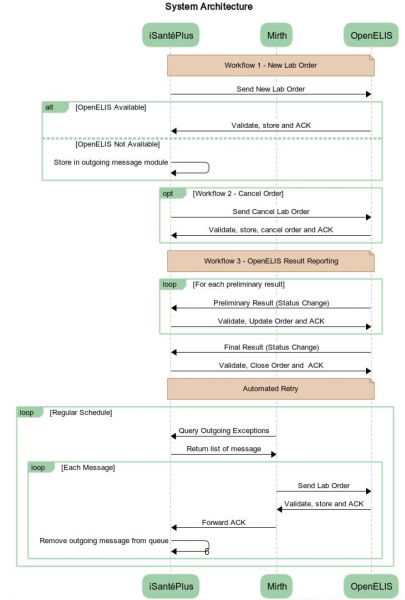
- Health Information Exchange (HIE) Bridge, modeled on OpenHIE: More complex than a direct bridge between OpenMRS and OpenELIS, this requires multiple pieces of a national eHealth architecture to be in place and supported. This solution offers flexibility for addressing additional and more advanced use cases for data exchange between OpenELIS, OpenMRS, and other systems, such as a national aggregate health indicator reporting system (e.g. DHIS2) or a master health facility list or a terminology service. A technical description of this strategy is shown in Figure 3.
Figure 3: Technical Description of OpenELIS – OpenMRS Bridge via OpenHIE
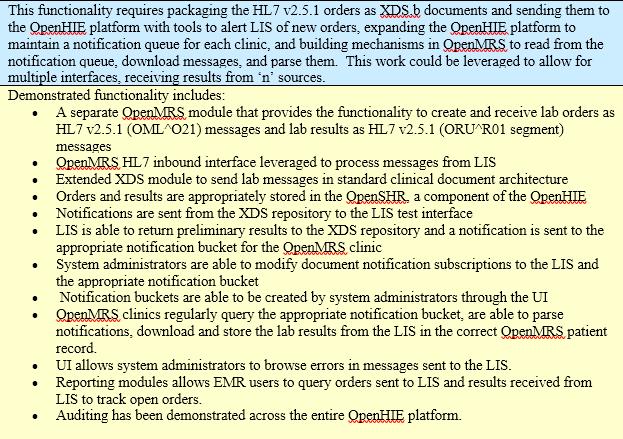
Both strategies will build upon I-TECH’s foundational work with LIS - EMR interoperability in Haiti, having developed both a direct bridge prototype and an OpenMRS-LIS data exchange deployment using OpenHIE. In 2017, I-TECH re-developed the Haiti national EMR, iSante, using the OpenMRS platform, gaining substantial experience with the OpenMRS development and integration with the OpenHIE interoperability framework. The goal of redeveloping the system using the OpenMRS platform is to enhance sustainability of the system by leveraging a well-established global digital good with a broad developer and implementer community. This proposal would expand on that goal by contributing more advanced functionality and integration for the broader global goods community.
The current proposal will build upon the existing Haiti work to generalize the solutions such that they can be robustly implemented in other instances where OpenMRS - OpenELIS interoperability is desired, either through a direct bridge or through OpenHIE. We specifically propose to design and develop generalized prototypes for these two solutions via the following activities:
- Activity 1.1: Document functional and technical specifications for OpenELIS-OpenMRS workflows, including patient identification and registration, lab test orders, and lab test results;
- Activity 1.2: Generalize the technical designs for both the direct bridge and the HIE bridge type solutions;
- Activity 1.3: Develop generalized minimum viable products (MVPs), provide testing and quality assurance, and release the products;
- Activity 1.4: Develop documentation of both implementation and technical aspects of the MVP releases;
- Activity 1.5: Disseminate information about the MVPs with broader eHealth community through OpenMRS and OpenHIE annual meetings.
The goal of Activities 1.1 and 1.2 will be to generalize functional and technical specifications for both the direct bridge and the HIE bridge developed under I-TECH’s Haiti project, and then work with the OpenMRS community to review and refine the designs. The team will seek to modify the existing specifications so that anyone can leverage them in a variety of contexts and eHealth architectures. During the product development Activity 1.3, the UW team, with participation from both I-TECH and CIRG, will assign staff software developer who is familiar with OpenMRS and OpenELIS to meet the consensus specification for both the bridge and OpenHIE solutions. This work will result in two MVPs which can be tested in a demonstration environment and suitable for implementation.
In Activities 1.4 and 1.5, the UW team will develop documentation of the releases which could be shared with developers, implementers, and decision makers. Documentation will describe the technical architecture and codebase as well as the requirements and workflows for successful implementation. To ensure that the work is widely shared and disseminated, we propose to present at least 2 webinars on the work and also to travel to attend the annual OpenMRS and OpenHIE implementers meetings in 2019 to present and build support for the work. Presence at these forums will be instrumental in developing momentum toward wider deployment, gathering of lessons learned, and progressive future improvement upon the MVPs.
As a final step within the scope of the presently proposed project, the consortium team will seek to identify implementation sites and partners which would be suitable to deploy the beta releases and gather feedback. Carrying out production-level deployment of the two solutions in clinical and laboratory settings is beyond the scope of this proposal but could be undertaken in a future phase. Also part of future phase activity would be publishing and sharing a prioritized roadmap in the OpenMRS, OpenLIS, and OpenHIE communities of practice for improvements and additional features, based upon what is learned during one or more future deployments.
Workstream 2: Merging the OpenELIS Global and Bahmni OpenELIS Code Bases
The Bahmni software is a distinct distribution of the OpenMRS platform for hospital management, which includes an early fork of OpenELIS v3.1 from 2013. The Bahmni version of OpenELIS includes the basic functionality of lab management, but was customized through 2015 with additional solutions to lab workflows and non-standard interoperability with the other components within Bahmni. The main branch of the global OpenELIS codebase now includes much more advanced functionality for managing laboratory workflows and the information within, which have not been merged into the Bahmni codebase.
An example of some features presently available in OpenELIS Global which are not present in Bahmni include:
- Module for real-time UI based test catalog management module for modifying tests and test panel configurations; which ITECH has found to be a critical level of control for laboratory leadership to successfully implement and sustain OpenELIS in LMIC laboratories.
- Barcode label generation and batch entry capability to make it easier to process a high volume of orders.
- HIV viral load dashboard for visualization of population-level health outcomes.
- Easy install process, including a default test catalog.
For several years, the Bahmni developers have expressed a strong desire to move to the current OpenELIS Global code base to leverage the more advanced features, contribute to a community product, and to collaborate on shared roadmap features. However, Bahmni OpenELIS customizations have prevented the work from being addressed. Merging the two code bases within Bahmni will help to unify the OpenELIS community around an optimized software product and will support broader engagement of teams across the OpenELIS community who can then work on a forward-looking shared roadmap, ensure technology upgrades, and improve upon OpenELIS system features within Bahmni. In short, a shared code base will support long-term sustainability of the OpenELIS product by broadening the pool of developers to collaborate. For Bahmni, merging back into the main OpenELIS Global code base will allow Bahmni to leverage existing improvements as well as ongoing work happening under the OpenHIE LIS COP to strengthen OpenELIS.
The specific activities to be carried out by the consortium include:
- Activity 2.1: Assess differences between the two code bases, in both functional and technical aspects;
- Activity 2.2: Document functional and technical specifications for the software development team to merge the code bases;
- Activity 2.3: With the Bahmni Coalition, develop a roadmap to address the tasks for merging the code bases.
The roadmap for merging will identify high-priority, must-have development and will include steps for testing, quality assurance, and release. The UW team will collaborate with the Bahmni Coalition to develop and prioritize the OpenELIS roadmap via an in-person week long workshop and on-going virtual community collaboration. The workshop will include both design meetings as well as a developer hackathon to test and prototype portions of the code merging strategy. The UW team will then summarize the results of the workshop as a roadmap document which could be reviewed and commented by a broader set of Bahmni coalition members and members of the OpenHIE LIS COP before finalization.
Following completion of Activities 2.1-2.3, the OpenELIS community will collaborate with the Bahmni consortium to identify future resources and strategies to carry out the software development work. Other than preliminary test and prototype activities for designing the merge strategy, the software development actually addressing the merge will not be a part of this funding.
Workstream 3: OpenELIS and OpenLMIS Integration
Several countries, such as Cote d’Ivoire, Malawi, Tanzania, and Zambia, are implementing the OpenLMIS system to improve logistics data management and use. There are multiple use cases for integration of OpenLMIS and OpenELIS (see below) and such integration is of strong interest to VillageReach and I-TECH/UW. In collaboration with Village Reach, I-TECH plans to pursue integration of OpenELIS and OpenLMIS by mapping priorities for functional integration and by defining detailed specifications for product development.
Specific activities include:
- Activity 3.1: Review OpenLMIS and OpenELIS to identify preliminary user stories and use cases for integration of the products;
- Activity 3.2: Meet with lab professionals and other stakeholders from Haiti, Cote d’Ivoire, Vietnam, Cameroon, or other LMIC to gain input on use cases and functionality for LIS and LMIS interoperability;
- Activity 3.3: Define detailed specifications for interoperability to guide development, and develop proposal for level of effort and strategy necessary to achieve the work.
Activity 3.1 involves a discovery phase of collaboration between UW and VillageReach. As both organizations have active engagements with their user bases, we will seek to opportunistically leverage client input during the discovery phase. The outcome of this activity will be draft user stories and use cases, and draft high-level functional and technical specifications for LIS-LMIS interoperability. Then during Activity 3.2, UW and VillageReach will plan, organize, and sponsor a two-day workshop in order to obtain focused input from laboratory professionals from LMIC on the draft specifications. We will seek to convene a satellite workshop linked to the Global Digital Health Forum or OpenHIE Implementers meeting, and will sponsor laboratory professionals from at least two LMIC to attend the satellite workshop. The workshop will also be open to other interested attendees of the conference.
Following the participatory workshop, as part of Activity 3.3, the UW and VillageReach teams will use the input to refine and flesh out detailed functional and technical specifications. It is anticipated that the workshop will generate interest in executing the technical work necessary to achieve interoperability between OpenELIS and OpenLMIS, and may help to identify resources and resource persons for completing the work. However, the software development work goes beyond the current proposal and could be part of a later phase of work.
Use of Digital Health Technologies
Across the three workstreams, the UW team will collaborate with OpenMRS and OpenLMIS developers and implementers, including the OpenMRS community, the Bahmni Coalition, and VillageReach. Technologies which will be used during the proposed work include: OpenELIS Global v8.4 (https://github.com/openelisglobal/openelisglobal-core), OpenMRS, Bahmni (https://github.com/Bahmni/OpenElis), OpenLMIS (https://github.com/OpenLMIS), OpenHIE (https://ohie.org/), and Github.
OpenELIS is a standards-based open source laboratory information system that was initially developed by state public health laboratories in Iowa and Minnesota to support standard laboratory business processes as defined by the Association of Public Health Laboratories (APHL). It was forked and adapted into OpenELIS Global in 2009 by I-TECH and CIRG at UW to support both the basic and advanced clinical laboratory workflows in low-and-middle income countries. Since then, it has been continuously improved upon by multiple organizations to meet both a broader set of LMIC laboratory use cases and needs, and adapted by implementers for specific local and regional context. It has been implemented in Haiti, Cote d’Ivoire, Vietnam, Kenya as part of the national eHealth architectures, and is integrated as part of the core offering of the Bahmni HMIS distribution, used across multiple countries. In recent years, I-TECH has led software development and implementation of the global fork of OpenELIS, now in version 8.3. OpenELIS is built on a platform using Java, PostgreSQL, Tomcat, Struts, and Ubuntu 16, and I-TECH has plans to update the core product by moving from Struts to Spring (https://spring.io) in 2018-19 in order to ensure compliance with data security frameworks required in US government-supported laboratories.
OpenHIE is a community of practice which is dedicated to improve the health of the underserved through open and collaborative, development and support of country driven, large scale health information sharing architectures. The OpenHIE community seeks to enable large scale health information interoperability, offers freely available standards-based approaches and reference technologies, and supports community needs through peer technical assistance.
OpenHIE LIS Community of Practice (LIS COP) is a new open source sub-community of practice under OpenHIE that serves to coordinate efforts on several widely-used mature open source LIS products - OpenELIS Global, BLIS, Senaite (formerly Bika) an open source independent lab instrument interface software called OpenLabConnect, and the integration of these technologies into the broader facility-level and upper-level HIS ecosystem. Created in 2013, the OpenHIE collaborative has defined a comprehensive design pattern for the national eHealth architecture in low-and-middle income countries. The collaborative offers example reference applications, and health information standards to serve as the component or external system functions, with published implementation guides, defined standards, and other resources available.
OpenMRS is the most widely usedopen-source electronic medical record (EMR) system globally. It offers an open-source platform for building one’s own EMR, as well as a reference application for a “starter EMR.” The OpenMRS community has supported EMR implementations in thousands of health facilities globally, and brings together practitioners with different backgrounds including technology, healthcare, and international development.
Bahmni is a reference hospital information management system built as a distribution of the OpenMRS platform. It integrates the OpenELIS laboratory information system and Odoo ERP system. It includes modules for patient registration, clinical services, laboratory services, inpatient management, PACS integration for radiology services, stock management, billing management, and reporting.
Workplan and Schedule
The project is planned for a 12 month period. The workplan below lists tasks for each workstream and activity, and identifies who will be responsible (R), accountable (A), consulted (C), or informed (I). The workplan also shows the due dates for each deliverable as noted with “X”.
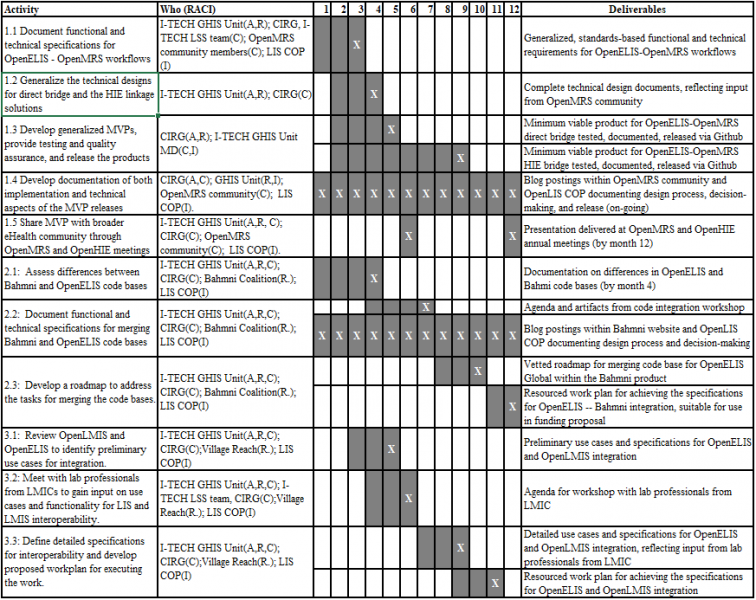
Project Deliverables
Workstream 1
- Generalized, standards-based functional and technical requirements for OpenELIS-OpenMRS workflows including: ordering a new laboratory test, canceling a laboratory test, sending a result, viewing a result, and reporting on tests ordered, tests canceled, pending tests, and results transmitted
- Minimum viable product for OpenELIS-OpenMRS direct bridge, which has been tested and documented and released via Github
- Minimum viable product for OpenELIS-OpenMRS direct bridge, which has been tested and documented and released via Github
- Presentation delivered at OpenMRS or OpenHIE annual meeting
- Blog postings within OpenMRS community and OpenLIS COP documenting design process, decision-making, and release
Workstream 2
- Documentation on differences in OpenELIS and Bahmi code bases
- Agenda for code integration workshop
- Vetted roadmap for merging code base for OpenELIS Global within the Bahmni product
- Resourced work plan for achieving the specifications for OpenELIS -- Bahmni integration, suitable for use in funding proposal
- Blog postings within Bahmni website and OpenLIS COP documenting design process, decision-making, and release
Workstream 3
- Preliminary use cases and specifications for OpenELIS and OpenLMIS integration
- Agenda for workshop with lab professionals from LMIC
- Detailed use cases and specifications for OpenELIS and OpenLMIS integration, reflecting input from lab professionals from LMIC
- Resourced work plan for achieving the specifications for OpenELIS and OpenLMIS integration
Digital Health Atlas
OpenELIS is registered as a project within the Digital Health Atlas.
Overview Summary
This set of global goods includes technologies for two-way data exchange between a leading open-source laboratory information system (OpenELIS) and two leading electronic medical record platforms (OpenMRS and Bahmni) as well as one logistics management information system (OpenLMIS,) so that diagnostic data for patient care and public health surveillance becomes more timely and accurate and laboratory systems function with minimum disruption in critical supplies. The requested investment from Digital Square will fund generalizable designs for message transfer between software products, deployment-ready products for linking LIS and EMRs, documentation and communication about the products and technologies.
Community Feedback
For Workstream 1, the UW team will elicit input from the OpenMRS community by posting to the talk forum, doing design forum sessions (leveraging the existing twice weekly design forum meetings), and building consensus with OpenMRS core developers on technical approaches, such as around handling the rest API. The project team will also collaborate within the OpenHIE LIS Community of Practice (COP) to ensure that the work is broadly relevant to LIS-EMR interoperability, and can be leveraged by development teams working with other LIS and EMR software products.
The UW team will support the activities of Workstream 2 by convening a face-to-face workshop focused on code merging, to include at least two developers identified from the Bahmni Coalition (possibly one from Leapfrog and one international developer). The UW team will also use video conference meetings and online chat channels such as Slack or Skype for daily to monthly communication with members of the Bahmni Coalition.
For Workstream 3, the UW and VillageReach teams, which are co-located in Seattle, will seek to work together through a series of half-day or day-long work sessions. As described above, the organizations will also collaborate to host a working meeting with laboratory-sector representatives from LMIC, as a satellite to a conference like the Global Digital Health Forum conference.
Use Cases and User Stories
Work Stream 1: Integrating OpenELIS and OpenMRS
Use Case 1: Clinicians need the ability to interface their EMR systems with LIS systems to automate many of the processes that deal with laboratory testing. This will reduce time and errors in testing and reporting. Clinicians need the ability to order and cancel tests from the EMR system and have those requests sent directly to the LIS. The LIS needs the ability to return test results and status to the EMR system. By integrating the LIS and EMR systems, these processes will be automated and will be available for viewing in both systems. This will decrease waiting time and increase efficiency and lead to better patient care.
Scenario: A Clinician is seeing a patient. She decides to order a lab test to diagnosis the patient’s medical condition. While in the OpenMRS system he creates a test request. This request is automatically transmitted to the OpenELIS system, which is deployed on a shared server within the clinic. The lab technician views the request and is notified by OpenELIS that the lab is out of the needed supplies to perform the test. An automatic notification is sent from OpenELIS to the OpenMRS about the stock out. The clinician requests the test be sent to another lab, the request is returned automatically to OpenELIS system.
Use Case 2: Many facilities require the same automated functionality around lab testing between their EMR and an offsite reference laboratory where the EMR and LIS systems are no co-located. In order to meet this need an automated linkage can be developed via the HIE. The tools will be developed that will allow the EMR and LIS to exchange message types via a third software interface that sorts and queues the messages and relays messages to both the LIS and EMR.
Scenario: A smaller hospital is using an EMR system but relies upon an external reference laboratory for certain advanced diagnostics, such as PCR-based tests. The lab orders and bio-samples are sent to the regional reference laboratory. Since the systems are not connected, this is a manual process for requests and outcomes. Using the linkage to the HIE, clinicians in the hospital now have a direct information sharing link to the regional reference hospital. As test requests are entered into the EMR system they are managed through the HIE interface and then delivered to the LIS system in the regional reference laboratory. Results are returned the same way, thus increasing efficiency and accuracy.
Workstream 2: Merging the OpenELIS Global and Bahmni OpenELIS Code Bases
Use Case 3: Hospitals across the globe are using the Bahmni package that includes an outdated version of OpenELIS. For ease of installation, better security, and more robust features these facilities would benefit from incorporating the latest features of OpenELIS Global v8 developed at UW. With the integration of OpenELIS v8 into the Bahmni platform the package will contain a robust and secure LIS system. This will allow greater flexibility in test management and reporting in laboratories. The integration will also increase cooperative work between the UW and the larger OpenELIS Global community. This will lead to greater efficiencies as a single code base will be in use and development will not be duplicated across multiple versions.
Scenario: A new facility is installing the Bahmni platform. They are opting for the full package that includes OpenELIS. They can be assured that they have the latest version of OpenELIS that meets current security and coding standards.
Use Case 4: There are currently multiple options around integration of LIS, EMR, and LMIS. By uniting the LIS COP, standards can be developed to create more streamlined documentation and installations. Standard and more detailed work plans around integration and use case scenarios will be developed and assist in the ease of adopting these systems on a larger scale.
Scenario: A regional hospital is installing OpenELIS as part of Bahmni. Installation is streamlined as there is one set of standards and procedures for installations. The technology team can easily find the latest version of the code base and the latest installation package, leading to a user-friendly implementation experience.
Workstream 3: OpenELIS and OpenLMIS Integration
Use Case 5: Without reagents, laboratory analyzers cannot process samples. When alerted to a reagent or test kit stock out, laboratory technicians can decide whether to perform the test using a different technique for which there are supplies or to refer the test order and sample to another laboratory which does have necessary commodities in stock. To be able to receive this alert the laboratory staff will need to be alerted in their LIS system of stock outs that are tracked by the LMIS system. Users will be able to order a test on a specimen that arrives in the lab, once the order is placed via the LIS system the lab staff would be notified by the LMIS system if there is a stock out of the needed supplies to perform the test. This will allow technicians to make a timely decision about what to do next. With this information they can determine if the test order should go to another facility or if the test can be performed using other items that are in stock.
Scenario: A clinician orders a test for a patient. The request is sent to the lab. The lab technician is immediately notified by the LMIS system that there is a stock-out of the needed supplies to test the specimen. The technician can notify the clinician that the lab does not have the needed supplies, the clinician can order a different test or ask for the test to be ran at a different lab. This will save valuable time in diagnosis
Use Case 6: Having granular data on usage of commodities at peripheral levels can help with forecasting and stock management at higher levels, to reduce both wastage and stock outs. When receiving commodity usage information for laboratory reagents and other commodities from laboratories in real time, it is possible to improve product tracking and forecasting within the national LMIS. Having this level of data will assist managers in understanding the demand at particular facilities and determine modify stock delivery based on demand.
Scenario: A regional commodities manager is noticing that a lab is consistently out of stock on supplies needed for a specific test. He can further see that a nearby lab is overstocked on the same supplies. The distribution system can be modified to reduce stock at one lab and increase stock at the other. This can be done in real time which will benefit both labs.
Self-Assessment on the Global Goods Maturity Model
A self-assessment of the OpenELIS software product is included as an Appendix.
Tags
- Laboratory information systems
- LIS
- interoperability
- OpenHIE
- Logistics Management Information System
- LIMS
- OpenLMIS
- Bahmni
- OpenMRS
Must be an existing global good as defined by
Must be deployed in at least three middle-income countries. (Please list the countries)
- Haiti, Cote d'Ivoire, Vietnam, Kenya, + Bahmni HMIS Distribution Implementations
Is it made available under Open Source Initiative approved software licenseThe software has been applied to a health domain?